Inside CoVent: How Krishna Dubba is Using AI to Revolutionise Event Sponsorships
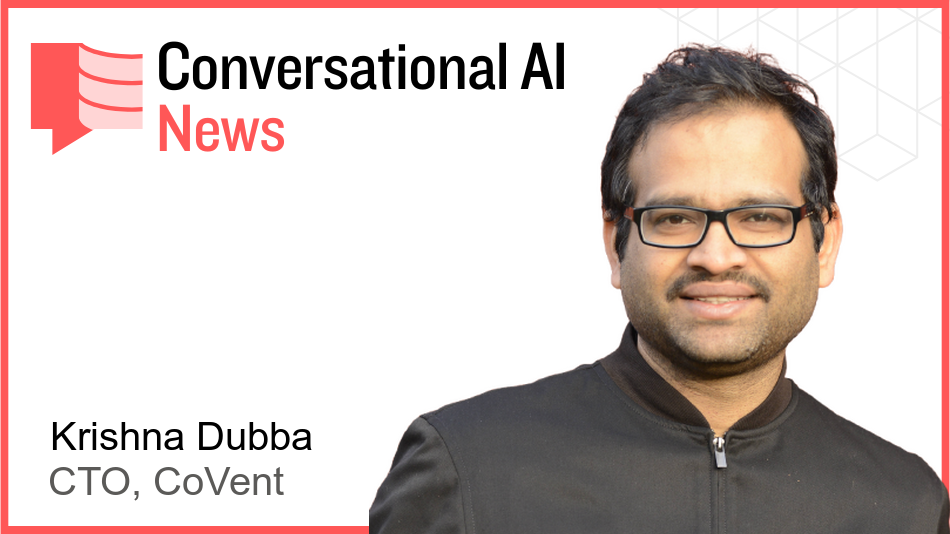
Today we're meeting Krishna Dubba, Co-founder and Chief Technology Officer at CoVent. They specialise in creating a dedicated marketplace for event sponsorships, connecting sponsors with event organisers.
Krishna knows a thing or two about AI (PhD in AI from Leeds) and takes a lean, cutting-edge approach to AI at CoVent. They're using open-source tools, multi-agent systems, and large language models to build scalable, adaptive solutions without relying on legacy infrastructure or heavyweight commercial platforms.
Check out his perspective below!
Over to you Krishna - my questions are in bold:
Who are you, and what's your background?
My name is Krishna Dubba, and I was born and raised in India. I hold a Bachelor's degree in Computer Science and a Master's degree in Artificial Intelligence from the University of Hyderabad, home to the only AI-focused postgraduate programme in India at the time (2004–2006).
Following my studies, I began my career at D.E. Shaw & Co., a global hedge fund, where I worked for a couple of years before moving to the United Kingdom to pursue a Ph.D. in Artificial Intelligence at the University of Leeds. During my doctoral and postdoctoral research, I specialised in cognitive vision, robotics, and knowledge representation and reasoning. I also contributed to several major research initiatives funded by the European Commission and DARPA. In the course of this academic work, I authored several peer-reviewed research papers and contributed to the advancement of the field through published findings.
Subsequently, I joined Nokia Advanced Research and later Bell Labs in Cambridge, UK, where I worked on cutting-edge projects involving computer vision, natural language processing, and deep learning. My transition into product-oriented R&D began when I became a founding member of Blue Prism's AI Labs, where I helped launch enterprise-grade features and products in the field of Robotic Process Automation. My work has led to multiple granted patents across various areas of AI and automation.
Building on this experience, I went on to establish startups focused on well-being and enterprise AI tools.
What is your job title, and what are your general responsibilities?
I am the Co-founder and Chief Technology Officer of CoVent, where I lead the company's technology strategy, oversee engineering teams, and drive end-to-end product development. Drawing on my background in AI research, enterprise automation, and deep tech innovation, I am responsible for shaping the technical vision and ensuring that our solutions are scalable, robust, and aligned with both market needs and long-term business goals.
Can you give us an overview of how you're using AI today?
We leverage artificial intelligence across multiple aspects of our platform:
- Content and behaviour-based recommendations and search to personalise user experience and surface relevant opportunities.
- Multi-agent systems for large-scale data scraping, extraction, and enrichment from diverse web sources.
- Large language models (LLMs) based custom reporting.
- Active research and development in causal AI and automated negotiation, exploring next-generation capabilities for decision-making and interaction.
Our platform is the only dedicated marketplace for event sponsorships, enabling sponsors and event organisers to discover collaboration opportunities, communicate and negotiate terms, execute contracts, manage payments, and access reporting, all within a single integrated environment.
As a young and agile startup, we have taken a modern approach to data acquisition and processing. Instead of relying on traditional, resource-heavy pipelines, we built our data infrastructure using cutting-edge technologies, including large language models (LLMs) and multi-agent architectures. This approach has allowed us to scale rapidly and achieve a level of performance comparable to more established competitors, despite operating with significantly fewer resources and in a shorter timeframe.
By bypassing legacy methodologies and embracing a forward-looking AI tech stack, we have positioned ourselves to deliver intelligent, scalable solutions in a highly dynamic market.
Tell us about your investment in AI? What's your approach?
We do not have a dedicated AI team; however, our engineering team possesses substantial experience with machine learning and large language models (LLMs). The systems we developed were built in-house, leveraging a wide range of off-the-shelf and open-source components, including multi-agent frameworks, pre-trained models, database extensions, and development SDKs.
Due to our unique requirements, commercially available, ready-made solutions were not suitable. Fortunately, the rapid proliferation of open-source tools and the emergence of numerous startups offering modular AI technologies have made it feasible to assemble tailored systems relatively quickly. While this approach is well-suited to agile startups, larger enterprises, often constrained by complex regulatory and compliance obligations, may find it more practical to procure comprehensive, vendor-supported AI solutions aligned with their specific use cases.
What prompted you to explore AI solutions? What specific problems were you trying to solve?
Traditional approaches to web data extraction are often time-consuming, resource-intensive, and costly to scale. As a startup operating with limited time and engineering capacity, we found that established workflows, especially those relying on conventional, template-based scraping, were not viable for our needs.
Our objective was to extract structured event data from millions of diverse and constantly changing websites across the web. Each event website tends to have its own unique layout, content structure, and interaction patterns, making rule-based or manually templated scraping highly inefficient and unsustainable at scale. While larger incumbents may have the resources to maintain massive teams or invest in highly specialised pipelines to address this complexity, we needed an alternative path.
To meet this challenge, we adopted a multi-agent systems approach. This allowed us to rethink our data extraction stack from the ground up, shifting away from brittle templates toward more autonomous, adaptive agents capable of handling heterogeneity across websites. By leveraging open-source frameworks, pre-trained models, and scalable orchestration tools, we built a system that can intelligently interpret, extract, and structure event data with minimal human intervention.
This architectural shift has enabled us to achieve high scalability and operational efficiency without the overhead traditionally required. It reflects a broader trend toward intelligent, agent-based automation in data-driven applications, particularly valuable for startups that must balance ambition with resource constraints.
Who are the primary users of your AI systems, and what's your measurement of success? Have you encountered any unexpected use cases or benefits?
CoVent is a dedicated marketplace that connects sponsors with event organisers. Our enriched, structured data provides valuable insights to sponsors (key stakeholders on our platform) enabling them to make well-informed decisions that drive measurable business impact. Through our advanced recommendation and reporting engine, both sponsors and organisers can discover relevant partnership opportunities, foster strategic collaborations, and build sustainable, mutually beneficial commercial relationships.
What has been your biggest learning or pivot moment in your AI journey?
Having spent a significant amount of time in the field of artificial intelligence, almost two decades, I've witnessed several technological waves rise and recede. At some point, Support Vector Machines dominated the landscape, followed by the prominence of Bayesian Networks and Convolutional Neural Nets, then the surge of Deep Learning, and now the era of Transformer-based large language models.
One key lesson from this progression is that there is no definitive or final technology in AI, what appears groundbreaking today may be eclipsed tomorrow. It is therefore essential to remain open to emerging, even currently underwhelming, ideas and not commit exclusively to any single paradigm. This mindset is particularly important for research labs in universities and large corporations, which are uniquely positioned to explore novel directions without the immediate pressure of commercial viability.
How do you address ethical considerations and responsible AI use in your organisation?
At CoVent, we take a holistic approach to responsible AI, focusing not just on our users but also on the impact AI has on our employees. We design AI systems with transparency, fairness, and accountability in mind, ensuring that automated decisions are explainable and aligned with both ethical standards and user expectations. Data privacy and security are core to our development practices, with minimal reliance on personal information.
We also recognise that AI can rapidly shift the value of certain skills, potentially displacing traditional roles. To address this, we actively invest in upskilling our team, providing training and encouraging continuous learning so employees can stay ahead of technological change and thrive alongside evolving AI tools.
By embedding ethical principles into both our product design and workplace culture, we strive to build technology that is not only innovative but also inclusive, responsible, and sustainable for everyone it touches.
What skills or capabilities are you currently building in your team to prepare for the next phase of AI development?
Our focus extends beyond simply learning new tools and technologies, we are also cultivating a mindset shift in how we approach and integrate AI into our work. The adoption of AI is not just a technical upgrade; it represents a fundamental transformation in how we think, operate, and design products and workflows.
AI is poised to redefine not only the tasks we perform but also the way we approach problem-solving, decision-making, and collaboration. To fully harness its potential, it's essential to rethink traditional work structures and processes, adapting them to leverage AI's strengths rather than retrofitting old models. This involves fostering a culture of experimentation, continuous learning, and openness to change, essential ingredients for thriving in an AI-driven future.
If you had a magic wand, what one thing would you change about current AI technology, regulation or adoption patterns?
If I had a magic wand, I would address the AI field's heavy dependence on CUDA, the programming framework tied to Nvidia GPUs. This reliance effectively concentrates much of AI model training and execution within Nvidia's ecosystem, driving up costs and significantly limiting hardware choices for developers and organisations.
While other hardware vendors like Intel and AMD have produced competitive chips, they haven't kept pace in delivering the accompanying software layers necessary for broad AI adoption. This highlights a critical lesson in the AI space: hardware alone isn't enough. Robust, developer-friendly software frameworks are equally essential for ecosystem dominance.
Reducing this dependency would open up the market, foster greater innovation, and make AI infrastructure more accessible and cost-effective for everyone.
What is your advice for other senior leaders evaluating their approach to using and implementing AI? What's one thing you wish you had known before starting your AI journey?
We're in an era of rapid acceleration and disruption, where waiting things out or relying solely on incremental change is no longer a viable strategy. Today's leaders must shift from a reactive to a proactive mindset, anticipating change, taking calculated risks, and being willing to experiment. The biggest risk now is standing still.
To stay competitive, it's crucial to engage with experts, monitor emerging technologies closely, and foster a culture that encourages small pilot projects or even internal hobby initiatives. These low-risk explorations often spark breakthrough innovations and build internal readiness before trends go mainstream.
Equally important: when evaluating solutions, don't default to the big, established players. Give startups a fair shot. Often, startups offer leaner, faster, and more customised solutions that are better suited to your specific needs, along with a level of customer support and drive that larger vendors simply can't match. While large SaaS platforms and consultancies may offer scale, they frequently deliver generic, slow-moving solutions that may not keep pace with your ambitions.
The future belongs to agile teams, sometimes fewer than ten people, who can move fast, stay laser-focused, and flip entire markets. Meanwhile, customer expectations are evolving rapidly. They're increasingly intolerant of mediocrity and willing to try more responsive, innovative alternatives. If you're not advancing, you're falling behind.
What AI tools or platforms do you personally use beyond your professional use cases?
We use tools like Cursor, Clause 3.7 Sonnet, Builder.io, and Bolt.new to accelerate our product development process. Additionally, Google's Agent Development Kit (ADK) supports our work in building intelligent, autonomous agents.
What's the most impressive new AI product or service you've seen recently?
From the beginning, I've been a visually oriented person, likely influenced by my background in cognitive vision research during my PhD. The recent advancements in text-to-video generation are truly remarkable. Tools like Google's Veo-2 and RunwayML's Gen-4 showcase just how far the technology has come. Even with a solid understanding of the underlying models, I still find myself in awe of the realism and creative potential these systems now offer.
This wave of innovation is set to transform and democratise rich content creation, lowering barriers for individuals and organisations to produce high-quality visual media in ways that were previously unimaginable.
Finally, let's talk predictions. What trends do you think are going to define the next 12-18 months in the AI technology sector, particularly for your industry?
One significant shift on the horizon is the rise of small, task-specific language models tailored for particular applications. These models offer efficiency and performance advantages in scenarios where large general-purpose models may be excessive or impractical.
I also anticipate growing adoption of Causal AI, which has the potential to become a mainstream component of data science workflows, particularly in decision-making and forecasting.
Another area poised for renewed interest is Automated Negotiation. Although the concept has been explored for over two decades, recent advances in large language models are breathing new life into this field, enabling more sophisticated and context-aware negotiation agents.
Thank you very much, Krishna!
Read more about Krishna on LinkedIn and find out more about CoVent at https://www.joincovent.com/.