A Personal Opinion on AI Implementation for Financial Services
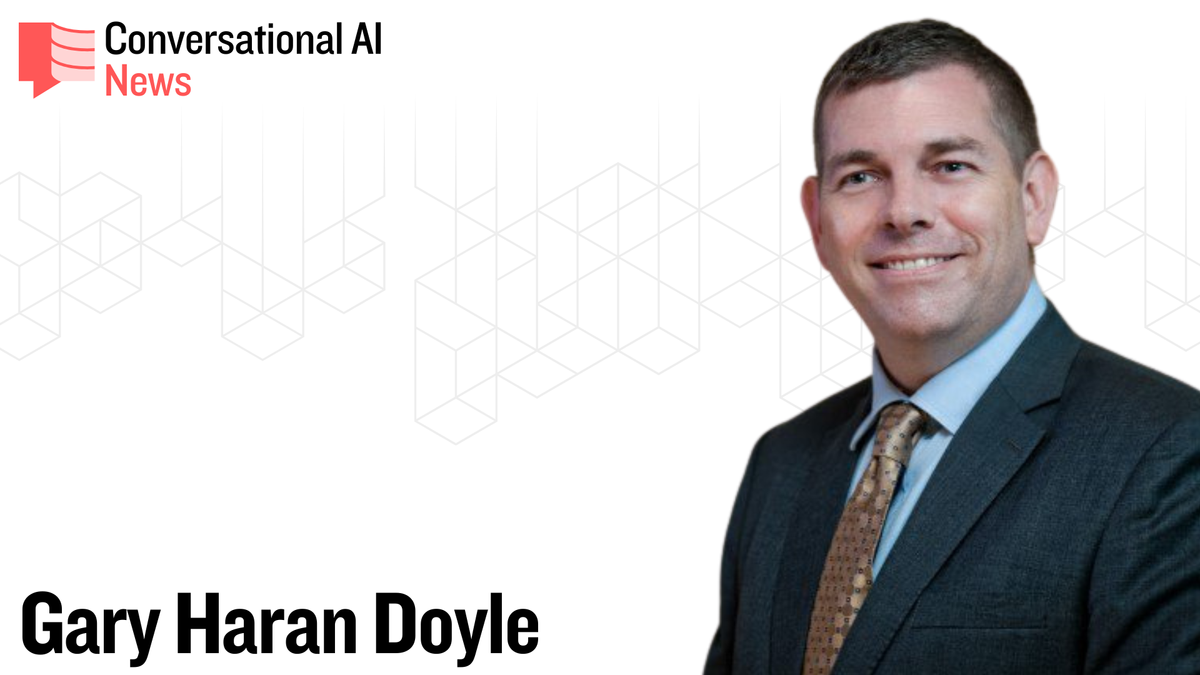
This groundbreaking technology of AI has prompted a desire at board level to make an impact using AI in financial institutions.
From HBR to PwC to The Economist, there are articles reminding board leaders that they need to be all-over AI to remain relevant. I’ve probably been somewhat guilty of supporting this, given my recent experience in Big Data, AI, Machine Learning, and Natural Language Processing at two successful suppliers to the Financial Services Industry (FSI), so I thought I would put down a few points which might be of interest.
AI is a broad catch-all term that includes Machine Learning (ML), Natural Language Processing (NLP) and Generative AI (GenAI). The first two are well known but GenAI (as seen in ChatGPT) is worth calling out as it exists to create new outputs. So, whilst you might use ML to screen customers at onboarding, or NLP to run a chat-bot, GenAI allows you to generate actionable insights at say, customer acquisition, or procurement, based on historic trends, demand profiles and past performance.
So, I thought I would take a little while to consolidate the groupthink from firms selling AI and services-around-AI and put together a quick, what the board should consider, list.
Do you have a Use Case? If not, find one. A small one.
What I found with the Big Data solution work that I did, was that more often than not, firms were let down by their data. Actually, let me correct myself here. ALL firms were ALWAYS let down by the quality of their data. Even the one’s which had just completed a $$$$$ Data Lake programmes. The lesson learned here (apart from “its never too soon to start a data lake programme”) is that an AI or GenAI project will unearth issues with your data, systems and processes, so start small and remember, that solving these might be expensive the first time, but your team and organisation will be better prepared for the next time.
Waiting for the perfect use case is not a good idea, because your first project will take longer and be more expensive than expected, its best to get something under the belt and have a big lessons-learned folder for the time when you want a big AI project and have no experience. This also means ensuring that the project knowledge remains within the organisation though using professional services to support your journey is also probably money well spent.
Prepare the Organisation, but don’t oversell it.
ChatGPT (GenAI) launched in November 2022, it took 3 months to collect 100m users and about the same number of news articles, employees were going to be fired, our children weren’t going to get jobs and James Cameron was right about killer robots all along... Consequently, your organisation may include some nervous employees, so a good place to start is with a project that makes their lives easier. I’m an experienced Banker and the job that I hated the most was anything that involved the 4-eyes principle. So perhaps AI that replaces that would be a good place, another place that gets a lot of turn-over of staff is the needle-in-the-haystack reviewing of Fraud and Money Laundering Alerts. Solutions for the 1st Line of Defence exist already and have proven successful, which brings me to my next point:
When is software really using AI?
There’s a lot of firms out there that have retrofitted AI to their existing product, now I don’t want to sound mean, but anyone that has implemented big software solutions into banks can tell you that is a tough job, careers are made and lost in the effort and not just of the IT dept., commissioning executives “just want it to work” and they seldom do. The consequence of my reminding you of this, is that just because someone has built some AI onto their existing software, doesn’t mean its going to deliver AI-value results. If you want AI, buy a dedicated AI solution. I heard this example recently “Adding AI to a Mercedes is not hard, having the AI work the Mercedes and the Autobahn together is very hard. Imagine if they had started with the AI”. Lastly, consider that a built from the word go AI (and GenAI particularly) solution is going to be more flexible around your organisation – more on that in the next couple of paragraphs.
Where can I put some AI in my firm?
As you know, financial services firms like to move in lockstep where possible, regulators like it that way and there’s less risk to the organization, and the leadership. But there’s also the Fear Of Missing Out and you’ve got to start somewhere. One of my favourite lessons-learned from decades ago, was about the power of group think, it’s the ability to ask everybody what their issues were, I used this shamelessly to get project-buy-in, but it was also close-to-my-heart as I too had once been a clerk slaving away at something menial wondering why we just don’t do ABC and then the world would be so much easier. Staff at all levels of your organisation today are experimenting the GenAI and the right questions from your Head of Innovation could generate projects with meaningful savings, or profits, depending on the role of whomever they ask. Incidentally, there’s a product for this, that uses AI to ask the initial and follow up questions in any language, please reach out if you would like to know more.
I’ve got a toe hold, am I ready for the big time?
The reason you got a toehold in the first place is prepare for the next project, whether that is another toehold, or a large project is up to you but I would like to suggest that an overarching reason for becoming an AI financial services firm is to ready to identify and mitigate a future risk and or take advantage of an opportunity. Not to use the earlier Mercedes analogy from an earlier couple of paragraphs too much, but being able to brake before the accident in front of you occurs is a feature of AI, as well as knowing that you can overtake safely. If you are stuck thinking “where do I need AI” perhaps you could instead consider this “now I have AI where is it best deployed today” and ask yourself that every day. Such an approach fosters an environment conducive to continuous learning and adaptation, enabling organizations to harness the full capabilities of this emergent technology.
Conclusion.
Start soon, start small, focus on the internal processes and pain points before building your killer robots solution. Use dedicated AI software that was built from the ground up to do AI stuff. Use external consultants sparingly. Remember it’s a journey and remember to ask your employees.
Gary Haran Doyle is a Banker turned Consultant, you can reach out to him on LinkedIn or at his email address.
If you'd like to contribute an opinion piece here on Conversational AI News, please email Ewan here or contact him on LinkedIn.